Averaging across all students who start college, only about six in 10 will be at that same institution by the next year.
More than a third of college students transfer to another school.
Why do so many undergraduates leave?
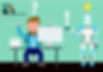
Colleges and universities work enormously hard to get the attention of prospective students, to drive up enrollments of qualified applicants and then have as many of those admitted as possible choose the school.
For tuition-dependent institutions, low retention rates carry enormous financial implications. Not only is a failure to persist bad for the student (particularly if they don’t transfer but stop attending), it is bad for the school.
This is where artificial intelligence will matter.
There is so much hype and worry about AI that it is hard to get a clear picture of what this technology will mean for higher education. What is important is to distinguish between general AI and narrow AI.
General AI is what Elon Musk is so worried about in his concerns that we are “summoning the demon,” and what has Ray Kurzweil so excited about the singularity. General AI is an all-purpose technology that will match and then surpass the human brain. Kurzweil thinks this will happen by 2045
.
Higher education people should forget about general AI. At least for now.
The action is in narrow AI, a technology that also goes by the name of deep learning. The big advances in AI over the past decade have all been in deep learning. This is the ability to predict or recognize a narrow outcome based on a narrow domain, a concrete goal, a robust algorithm and, crucially, massive amounts of data.
Deep learning algorithms can turn data into decisions. These decisions involve pattern recognition and prediction in a narrow domain. Narrow AI is what self-driving cars and speech recognition depend on. It is also narrow AI that is changing how highly repeatable processes such as the decision to offer credit or extend a loan are being made.
Narrow AI depends on massive amounts of data to optimize around a specific goal. Rather than being given a complete set of rules to follow, narrow AI applications depend on finding correlations and connections between vast numbers of data points.
Deep learning systems are not so much programmed as trained. The more data that are fed into the system, the better it gets at correctly identifying patterns, be they images or words or a borrower likely to default on a loan.
How does narrow AI relate to the problem of retention?
Colleges and universities have many problems when it comes to predicting who is likely to drop out or transfer. We don’t have much in the way of warning that a student is at risk of not persisting.
Those warnings, if they should come, often do not get to the right person on campus who can intervene. And of the actions that schools take to help a student persist, we are not clear which interventions are most effective.
AI is set to change all that.
AI systems will begin to collect data on students from an astounding number of sources and then utilize that data to “learn” about which students are at risk of not persisting.
These warnings will come much earlier than is currently the case. The alerts will also be paired with recommended steps to help the student succeed and stay in school.
The reason that this will occur is that the data net related to student success is about to get much wider. The AI system will ingest data from traditional sources -- everything from grades to test scores to financial and family data.
What will be different is that the AI will also utilize data points that are currently lost to make its predictions and recommendations. These data will come from the massive digital footprint that every student creates. Captured data may include everything from attendance at university sporting events, entries and exits in residence halls, and the days and times that a student swipes for meals.
AI systems will not only incorporate academic data points such as grades but the continuous data that are created through participation in adaptive learning platforms and learning management systems.
Academic data will be combined with social data. A student's social connections in the real world, such as whom they go to the library with or grab a meal with at a dining hall, will be incorporated into the analysis. (This should activating your Spidey sense around student privacy, a point I will address below.)
What deep learning does that people can’t do now is to find correlations and connections in all that mass of data that aid in prediction and decision making. The connections are too complex to be programmed and, perhaps more worryingly, even to be understood. But they will be orders of magnitude more accurate than our current early-warning systems.
The next generation of campus AI systems will be able to tailor recommendations for driving up the probability of a student persisting at the institution to different people who can take action. There will be one set of recommendations for the RA, another for each professor. The adviser will get a set of recommendations, and so will the dean. These recommendations will be tailored to both the student and the recommender.
Every university president should consider herself the chief data evangelist of her school. Only the president -- or maybe the provost -- can provide the push that schools need to move to a data culture that will enable advances in AI to make meaningful changes in retention.
Forward-thinking institutions will understand that the most important assets they possess are data. They will translate this recognition into an institutionwide commitment to make data-driven decisions.
There are many tricky issues to be worked out concerning the privacy and ownership of student data. Students will need to know that everything they do that leaves a data trail will be utilized in the school’s AI student success systems. They should have access to and ownership of their data. The method in which their data are used to predict success should, to the extent possible, not be a black box.
Fortunately, this is also a teaching opportunity. Narrow AI, and the deep learning that powers it, is set to change many aspects of our economy and society.
Colleges and universities should be at the forefront of both using and teaching about this technology.
Higher education should be setting the standard for responsible data collection and usage.
We should be leading the way into our (narrow) AI future.
Some opinions expressed in this article may be those of a guest author and not necessarily Analytikus. Staff authors are listed in https://www.insidehighered.com/users/joshua-kim