Artificial General Intelligence (AGI) is the long sought after ‘brain’ that brings together all the branches of AI into a general purpose platform that can perform with human level intelligence in a broad variety of tasks. Will it free us or replace us? It’s closer than you think.
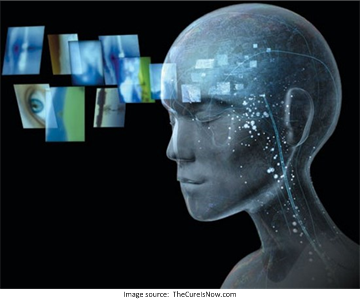
Can you see the forest for the trees? That’s one of the questions an AI system might be asked to answer and probably not succeed. However, if you’re trying to follow the general trends and literature in AI, we might ask ourselves the same question.
We are inundated with articles about advances in image processing, natural language (speech) and text processing (the big three of deep learning), plus robotics. But you may have missed the very important goal of blending these together into an Artificial General Intelligence platform
(AGI) which is the holy grail of AI.
To understand the AI you read about today you need to start by asking yourself, is what I’m seeing about Strong versus Weak AI and/or about Broad versus Narrow AI? All of AI’s incremental improvements can be characterized along these two dimensions.
Strong versus Weak AI
The Strong AI camp works on solutions genuinely simulating human reasoning. The Weak AI camp wants to build systems that behave like humans, pragmatically just getting the system to work.
Narrow versus Broad AI
Another division in AI development is Narrow AI versus Broad AI. Given that the requirement is that the machine perform the same task as a human then Narrow AI allows for lots of limited or narrowly defined examples, including outside of deep learning. For example, NEST thermostats or systems that recommend options (what to watch, who to date, what to buy). Broad AI then is a system which can be applied to many context sensitive situations in which it can mimic human activity or decision making.
The goal of Artificial General Intelligence (AGI) is to create a platform that is Strong (simulates human reasoning) and Broad (generalizes across a broad range of circumstances).
The Pursuit for AGI is Alive and Well
Not that much has been seen in the popular press recently about the pursuit of AGI. For starters, the incremental gains in partial AI represented by deep learning and robotics have bigger money and bigger companies behind them and more close-in applications. Nothing wrong with that. Economics dictates that we move forward in an incremental manner making profit from our efforts as quickly as possible so these advancements are pushed to the forefront of our attention. However, the pursuit of AGI is alive and well in academia, and also in some very interesting startups.
Deep Learning and Robotics are the Body – AGI is the Brain
One way to make sense of this is to see the elements of deep learning and robotics and the arms and legs of AGI, or more correctly the eyes (image processing), the ears and mouth (text and natural language processing), and the body (robotics, especially new haptic developments).
Now, like the scarecrow in the Wizard of Oz, if I only had a brain.
You don’t need to look far for examples of the limitations of today’s smartest systems. Take this exchange with Siri. Siri, who was the first President of the United States. Siri: George Washington. Siri, how old was he? Siri: 234 years. This answer because Siri could not recognize the context that I was asking about George and not about the United States. It’s in anticipating context where our AI systems mostly break down.
How Will We Know When We Get There
Since the discussion started in earnest in the 1950s, researchers have been proposing different tests of whether a system might be considered ‘intelligent’. We’ve all heard of the Turing test. Can a system fool a human being into believing that the human is interacting with another human. But there are more interesting and meaningful tests.
The Coffee Test (Goertzel)
A machine is given the task of going into an average American home and figuring out how to make coffee. It has to find the coffee machine, find the coffee, add water, find a mug, and brew the coffee by pushing the proper buttons.
The Robot College Student Test (Goertzel)
A machine is given the task of enrolling in a university, taking and passing the same classes that humans would, and obtaining a degree.
The Employment Test (Nilsson)
A machine is given the task of working an economically important job, and must perform as well or better than the level that humans perform at in the same job.
Certainly the last one seems most on point since it ties performance to economic achievement. However, it’s unlikely we could find a single human able to master every single economically important job so perhaps we can declare victory when the AGI can master one or more jobs if not all. It’s also important to note that were not asking for a system that is demonstrably conscious, self-aware, or moral.
To summarize, our AGI system will have to display human-like general intelligence not tied to a specific set of tasks. It must be able to generalize what it has learned especially to extend those generalizations into contexts qualitatively very different from what it has seen before
.
What Tools Would an AGI Platform Need?
This question has been at the heart of many AGI conferences and the answers are as numerous as the academic disciplines studying the question. But we can take a bit of a shortcut and talk about reasoning styles. The general purpose AGI platform would need all three deductive, inductive, and abductive reasoning.
Deductive reasoning starts out with a general statement or hypothesis and examines the possibilities to reach a specific, logical conclusion. The scientific method uses deduction to test hypotheses and theories. In deductive inference, we hold a theory and based on it we make a prediction of its consequences. That is, we predict what the observations should be if the theory were correct. We go from the general — the theory — to the specific — the observations.
Inductive reasoning is the opposite of deductive reasoning. Inductive reasoning makes broad generalizations from specific observations. In inductive inference, we go from the specific to the general. We make many observations, discern a pattern, make a generalization, and infer an explanation or a theory.
Abductive reasoning usually starts with an incomplete set of observations and proceeds to the likeliest possible explanation for the group of observations. It is based on making and testing hypotheses using the best information available. It often entails making an educated guess after observing a phenomenon for which there is no clear explanation.
It is abductive reasoning that helps us jump from one context to another and is the most often missed tool in the AI arsenal. You might say that the abductive reasoning capability we need would start with some sort of hypothetical scenario generator. Those hypotheticals would then be winnowed down to the best estimate or guess.
How Close Are We
Every AGI conference seems to ask this question and come to somewhat different conclusions. There is significant disagreement as to whether existing systems emulate a 3rd grader or a second year med student depending on who’s doing the evaluation.
The number you see pop up most often though is 2050. That’s the date that many leading investigators say we should expect to see robust AGI platforms in wide adoption capable of performing a variety of human tasks with the same or greater skill level as the humans they’ll displace.
35 years seems like a long time to wait. 35 years ago we were just seeing the emergence of PCs in the workplace and were still more than a decade away from the World Wide Web. So 2050 seems like a bit of a cop out. The limitations that exist are only engineering problems and like all engineering problems will eventually be overcome. Especially with the huge amounts of money being spent by our largest and smartest tech companies.
Still, slowly grows the tree of knowledge and this will be an incremental journey driven by academia, small startups, and especially by our largest tech companies.
IBM’s jeopardy playing Watson is an example of that direction. Watson looks at thousands of pieces of text that give it confidence in its conclusion. Like humans, Watson is able to notice patterns in text each of which represents an increment of evidence, then add up that evidence to draw the most likely conclusion. It’s said that Watson can now detect sadness in your writing.
I’m sure IBM is trying for many applications, but one that seems promising is for Watson to become the world’s smartest medical doctor with exceptional diagnostic powers. While that would be a remarkable feat, it still falls well short of a general purpose decisioning platform that can be used in multiple contexts.
In 2014 Google bested FaceBook in their pursuit to buy the company Deep Mind for more than $400 Million. This is a company that has no product and no revenue but was reported at the time to have about 25% of the world’s leading experts in AI (that’s 12 people out of a worldwide expert population estmated at only 50 in 2014).
Then there’s startup Beyond Limits which has agreements with NASA via JPL and CalTech to utilize their IP in AI. They are definitely in pursuit of an AGI platform but their immediate goal for monetization may surprise you. It’s making digital marketing more efficient. Even with the best targeting, click through rates on digital advertising look like 1:1000. And if you shopped for say a toy for a new born because you were going to the shower, you are likely to continue to get advertising based on that search for months and months to come. A context aware AGI platform would assemble a much larger body of data and become aware that this is not a repeating purchase desire, presumably making digital marketing several orders of magnitude more effective.
Job Propects
If you are already a data scientist you know you have the best job in America with a recently reported median base salary of about $117,000. However, if you read the part above that in 2014 there were only about 50 recognized leaders in AI in the world you might want to jump on that band wagon. A reliable source (but unconfirmed) recently told me that IBM, Google, and the other big tech players are literally sweeping clean the masters-level data science graduates at top universities with specialties in AI with starting salaries of $175,000 and no experience! If the timing and interest works for you, that is you’re just starting a Masters level program in DS, then a specialty in AI could lead not only to an extraordinary salary but also to a place in the pursuit of Articial General Intelligence.
Some opinions expressed in this article may be those of a guest author and not necessarily Analytikus. Staff authors are listed http://www.datasciencecentral.com/profiles/blogs/artificial-general-intelligence-the-holy-grail-of-ai